BronchoNAV
AI-based navigation support for bronchoscopic interventions
Video Bronchoscopy (VB) is commonly applied in conjunction with lung diseases. It is a fundamental procedure for diagnosis of lung cancer enabling biopsy of deep airway tissue. In addition to that, VB is routinely conducted for monitoring Chronic Obstructive Pulmonary Disease (COPD) patients and clarification of acute respiratory problems at Intensive Care Units (ICU). The navigation within the bronchial tree is challenging and physically demanding for physicians due to homogenous textures and perceptually similar appearance of bronchial orifices. This is particularly the case in the absence of prior CT scans and Electromagnetic Tracking (EMT) systems at ICUs. BronchoNAV investigates novel AI-based methods to enable support for physicians while navigating inside the bronchial tree using solely vision as input, omitting EMT and prior patient-specific CT scans. It enables real-time superimpositions of detected airway orifices supplemented with recognized anatomical airway labels (e.g. RB1, TriLuL etc.).
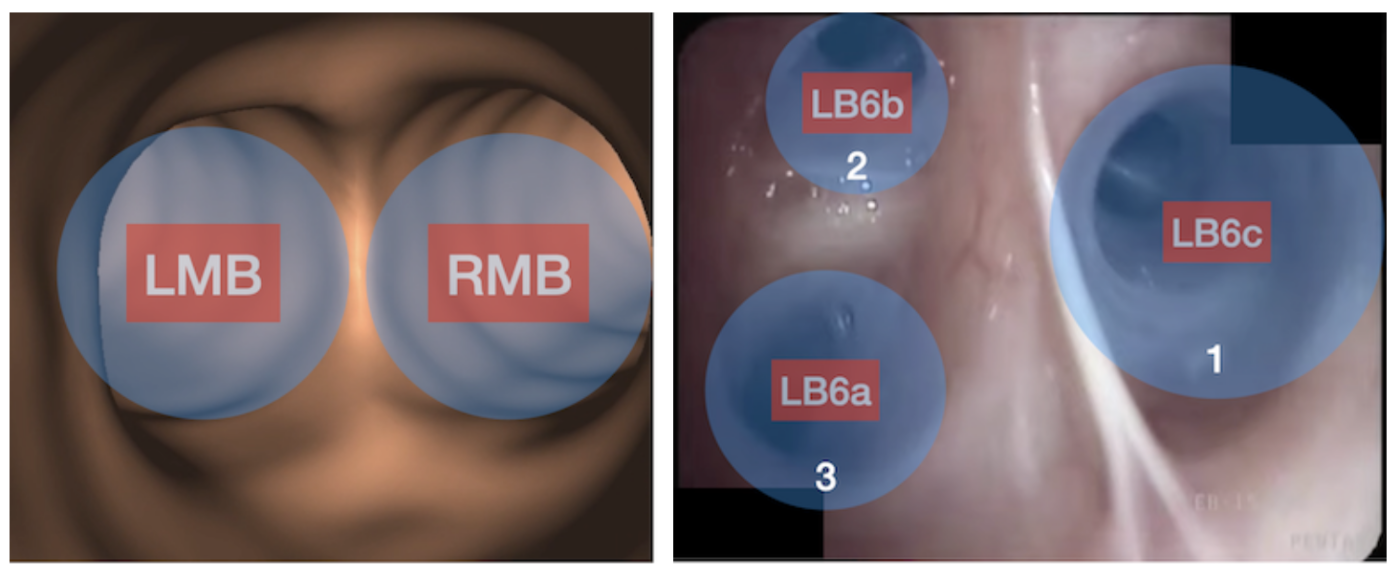
Multi-label segmentation of airway orifices is a key feature for supporting bronchoscopy interventions. By superimposing anatomical labels onto bronchoscopy videos we limit mental stress and raise confidence for physicians. The availability of large-scale CT scan databases leverages learning anatomical models which are combined with textural information from real bronchoscopy videos using deep learning-based methods.
Publications:
[1] Ron Keuth, Mattias Heinrich, Martin Eichenlaub, and Marian Himstedt: Weakly Supervised Airway Orifice Segmentation in Video Bronchoscopy. arXiv preprint arXiv:2208.11468 2022
[2] Fenja Falta, Lasse Hansen, Marian Himstedt, and Mattias P. Heinrich: Learning an Airway Atlas from Lung CT Using Semantic Inter-patient Deformable Registration. In Bildverarbeitung für die Medizin, 2022